enrollment
How campuses can use predictive analytics to focus college student recruitment more strategically
Data driven, data informed, metrics, analytics—it seems that, as enrollment managers, these terms are at the forefront of all that you do. I recently read an article in Inside Higher Ed that addressed the amount of data that colleges and universities are trying to understand and make decisions upon. The terms “big data” and “actionable analytics” were used to describe efforts to make data-informed decisions that help more students enroll, persist, and ultimately graduate.
We are big believers in data at Noel-Levitz. As my colleague Peter Bryant says, “track everything that moves and don’t do anything twice unless you know it works!” We’ve been giving this advice to campuses for years, and it seems that in today’s competitive and economically challenged environment, more and more campuses are in agreement.
To be effective, though, you have to take action with data. You have to not just track everything that moves, but understand what those data mean. There is one type of data that helps many of our client campuses enroll students much more efficiently: predictive modeling.
Predictive modeling for enrollment is an inherently action-oriented process. It takes your previous enrollment data, applies it to prospective students, and provides an assessment of each student’s likelihood of enrolling. (It also works for assessing the likelihood of current students persisting, but I’m going to focus on its admissions applications here.)
Campuses across the country are using this technique to run more efficient operations by focusing their limited resources on those students who are more likely to enroll. Let me illustrate how this works with two of the most critical elements of student recruitment: purchasing student names and qualifying inquiries. The following are based on millions of prospective students that our campus partners qualified through predictive modeling last year.
Qualifying list buys
One of our predictive modeling services allows you to qualify student names before you buy them. Every student receives a score from 0.00 (extremely unlikely to enroll) to 1.00 (extremely likely to enroll). For the fall 2011 entering class, 97 percent of the enrollments for our campus partners came from students with a model score of .6 or higher.
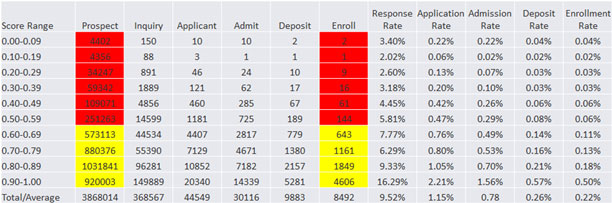
What does that mean? It means you can segment your search purchase and your communication flow by model score. I recommend the following:
- Purchase student names with high model scores. This allows you to buy names of those students who are more likely to enroll at your institution, eliminating potential waste by not buying students who will never enroll at your institution.
- Load the highest scoring names directly into your inquiry pool. Many of our campus partners will take a sub-set of their purchased list and treat them as inquiries. The value of the predictive modeling score is that you can accurately pinpoint which students are most likely to enroll.
- Segment your messaging by model score. Consider putting your search names into different buckets by score range. The highest model score students might receive the most robust communication flow (including written and electronic). Those with lower scores may receive a series of e-mail messages.
Inquiry pool qualification
The table below shows data from campuses qualifying their inquiry pool with our predictive modeling service. As you can see, 85 percent of the net deposits came from half of the inquiry pool.
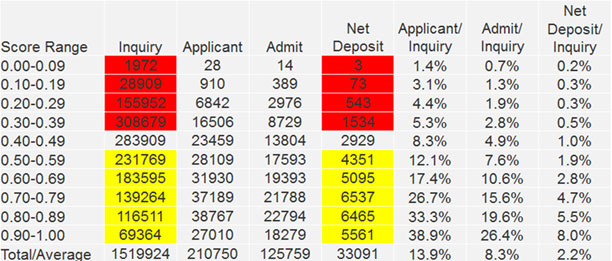
This kind of qualification means that schools can better focus their energy, effort, and resources on those inquiries who are more likely to enroll. With this type of actionable data, institutions can:
- Save money on expensive mailings. If students in the lower score bands will likely not enroll no matter what you do, why send them the expensive four color publications? It makes more sense to communicate with those students via e-mail instead.
- Build stronger relationships with prospective students. Predictive modeling allows each counselor to build a “hot prospect” list based on model score and then create a robust communication plan to those students.
- Travel smarter. Rather than visiting those high schools with the most inquiries, you can now visit those with the most qualified inquiries. In other words, your focus shifts from quantity to quality, potentially increasing your return on investment.
Here’s just one example of how you could use model scores to prioritize communication strategies:
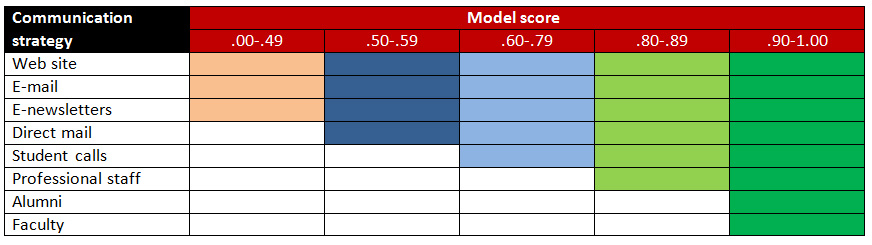
Notice that you still communicate with all the students in your pool. However, you allocate your resources much more strategically than you could have without predictive modeling.
Campus outcomes speak volumes. Think of your own pool and how it would help you to have this type of data at your disposal. Given the current economic climate and budget scenarios on many campuses, it is almost hard to imagine an effective enrollment plan that does not include predictive modeling.
I am going to discuss how predictive modeling benefits enrollment managers in more detail during a free Webinar on March 8. I highly encourage you to consider attending, and I will be happy to answer your questions during the Webinar. Or drop me an e-mail if you have any questions. Regardless, if you haven’t looked into predictive modeling for your recruitment efforts, I suggest you do so. It really provides a remarkable advantage for campuses.